Bringing a new ADAS/ AD system on the road is an expensive and often complex undertaking. Validating an autonomous driving function is a particularly large project. It requires huge amounts of perception data to be labeled at high quality. One of the biggest budget and time constraints is the manual part of the labeling process. Discover how an automotive Tier 1 supplier broke a speed record in producing image annotations for a development and validation PoC of a computer vision product.
Ambitious ADAS Development Timelines Driven by OEM Customers
The Proof of Concept project deadlines were driven by the development team’s ambition to present unprecedented development and validation times to their automotive OEM customers. The only way to reach the necessary throughput was to take an annotation supplier on board capable of highly automated tool chains.
Key Validation Project Figures
- Fully managed annotation service
- Annotation type: 2D bounding boxes, 7+ classes & attributes
- Annotation volume: 27,000 km with an estimated 20 million objects
- Annotation timeline: 12 weeks delivery time
- Annotation quality target: 98% Delivery: 24/7 operation with daily batch deliveries
The Annotation Supplier Challenge
There were several requirements including the experience in automotive, labeler supplier base and tools enabling good collaboration between the data annotation supplier and the internal QA team.
»But the main prerequisite was the automation capability as this was key to produce large amounts of annotations in a short amount of time while ensuring a high level of quality. Data quality is extremely important as it drives computer vision feature performance and confidence.«
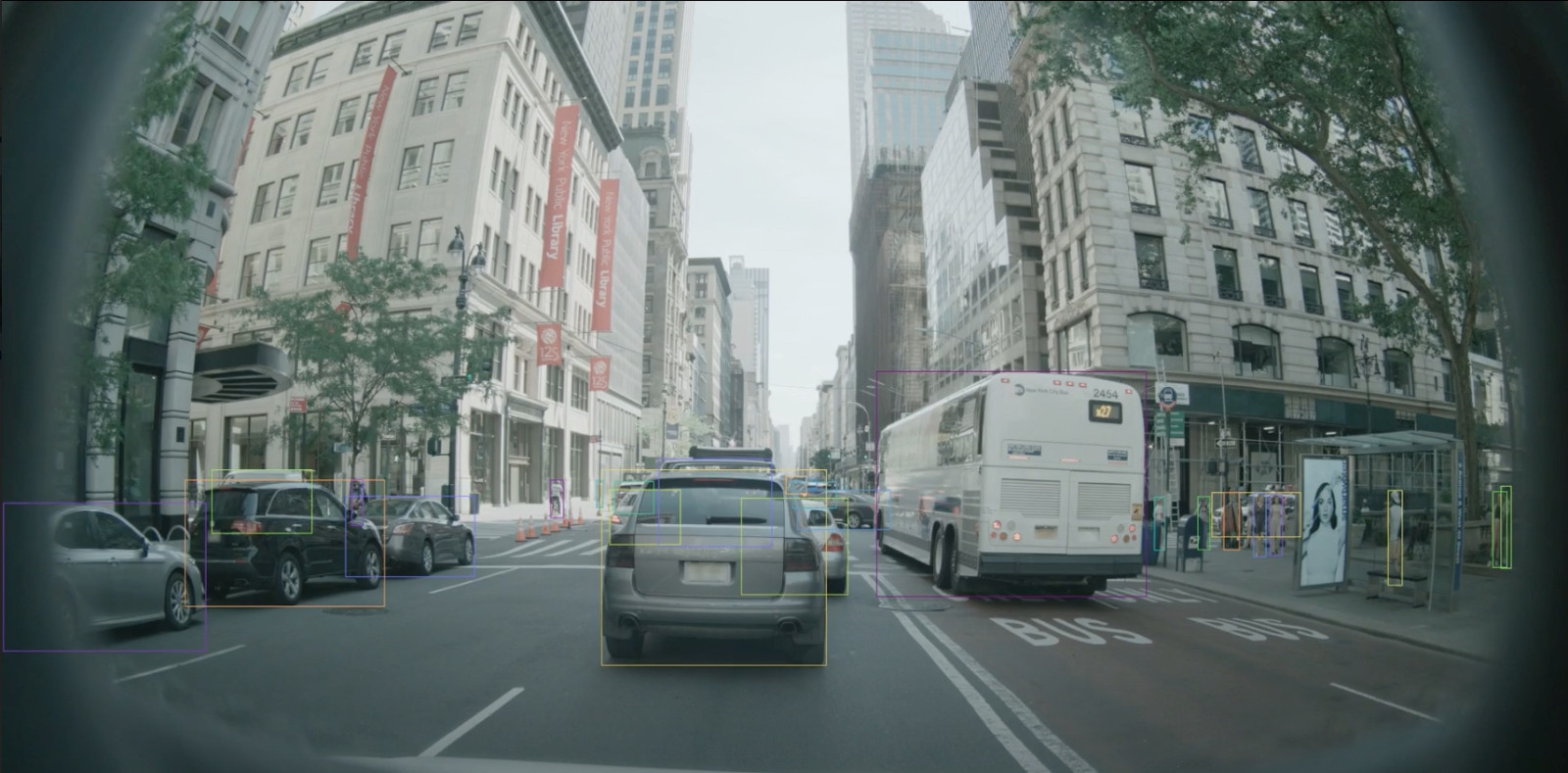
Well aware of the challenges of project managing large labeler teams, the project team opted in for a fully managed service. Even the most mature AI-powered technology is still depending on manual input to quality check the outcome of pre-labeling algorithms. understand.ai was selected for its competence in deep learning & other techniques needed to reach a high level of automation and the experience in working with Tier1s and OEMs in the automotive area. UAI was one of the 2 suppliers selected for the project. The 2 suppliers were benchmarked against each other to compare the ability to automate. Only understand.ai completed the project.
How to label 20+ million objects in 12 weeks’ time?
In a large scale annotation project, a lot can be achieved with a fast set-up and well orchestrated project execution. Even more can be done with quick onboarding of labeling service partners and an easy-to-use annotation tooling. Yet none of that would be enough. Automated annotation is essential. Moreover, the output of the pre-labeling algorithm has to be of high quality unless you want to lose the time saved with automation on manual quality checks. The project team tested UAIs capability to produce the required amount of annotations given the time-frame. They knew there were lead times involved to get the project going, for example, setting the labeling instructions and clarifying the handling of edge cases. They also knew that without high automation rates the annotation supplier wouldn’t be able to meet the deadlines.
Why Annotation Automation Matters
The UAIs machine learning model was continuously retrained with more data resulting in a 68% decrease in time to accepted annotation from beginning to the end of the project.
UAI delivered
- 23.4 mio objects in less than 12 weeks - 3.3 mio more than planned
- in peak throughput 1.4 mio object annotations per day, all accepted first time through
- the quality target of 98%
Let’s put that into perspective. Based on the improved average time to accepted annotation, the project team would need more than 22 weeks with the same size labeling team to complete the annotation of 23.4 million objects at the initial automation rate.
At this sample sequence you can see the object detection quality and annotation precision produced fully automatically. No manual rework was involved.
Why Automotive Experience Matters
Years of experience with large scale annotation projects for autonomous driving proved to be of equal importance for the success of the project as the machine learning competence.
- Having a common vocabulary and shared experience of common ADAS/ AD challenges saved time and prevented time consuming clarifications
- UAI experienced project management
- Facilitated a project set-up in record time
- Ran a 24/7 operations with daily deliveries
- Coordinated teams of 4 labeling partners throughout the project
- Maintained good customer handling in terms of transparency in reporting and flexibility throughout the entire 12 weeks.
- Having an ecosystem of experienced labeling partners proved to be extremely beneficial in 2021. UAI was able to rapidly deploy teams in geographic locations not heavily impacted by the Covid pandemic.
Opening New Possibilities for Autonomous Driving Validation
The development team of the Tier 1 has presented the Proof of concept result to its key OEM customers and received a very good feedback with respect to performance and functionality. The collaboration with understand.ai proved the feasibility of projects where thousands of kilometers have to be driven to prove that an autonomous vehicle perception AI is working accurately.