The concept of 'ground truth' has evolved beyond its conventional role, becoming a foundational term within Machine Learning and Deep Learning communities. In this discourse, 'ground truth' represents an impartial and objectively verifiable observation of an object's state—a factual reference.
In this article, we want to explore the pivotal role of ground truth labels when it comes to enhancing the effectiveness of Machine Learning models.
Core Dynamics of Machine Learning
In the diverse landscape of Machine Learning, you'll come across different methodologies and approaches such as supervised, unsupervised, and semi-supervised learning. The crucial link tying them all together is the essential role of ground truth labels. That is the case because Machine Learning operates like a 'black box’ - we assess its performance through practical measurement. This involves running the algorithm on new examples and comparing predictions with the ground truth. Consequently, no matter which method is employed, in order to ensure top-notch results for the test data, we require high-quality ground truth labels.
Without delving further into the intricacies of these various methods, it’s essential to point out that only supervised algorithms currently deliver the high-quality outcomes demanded by the industry.
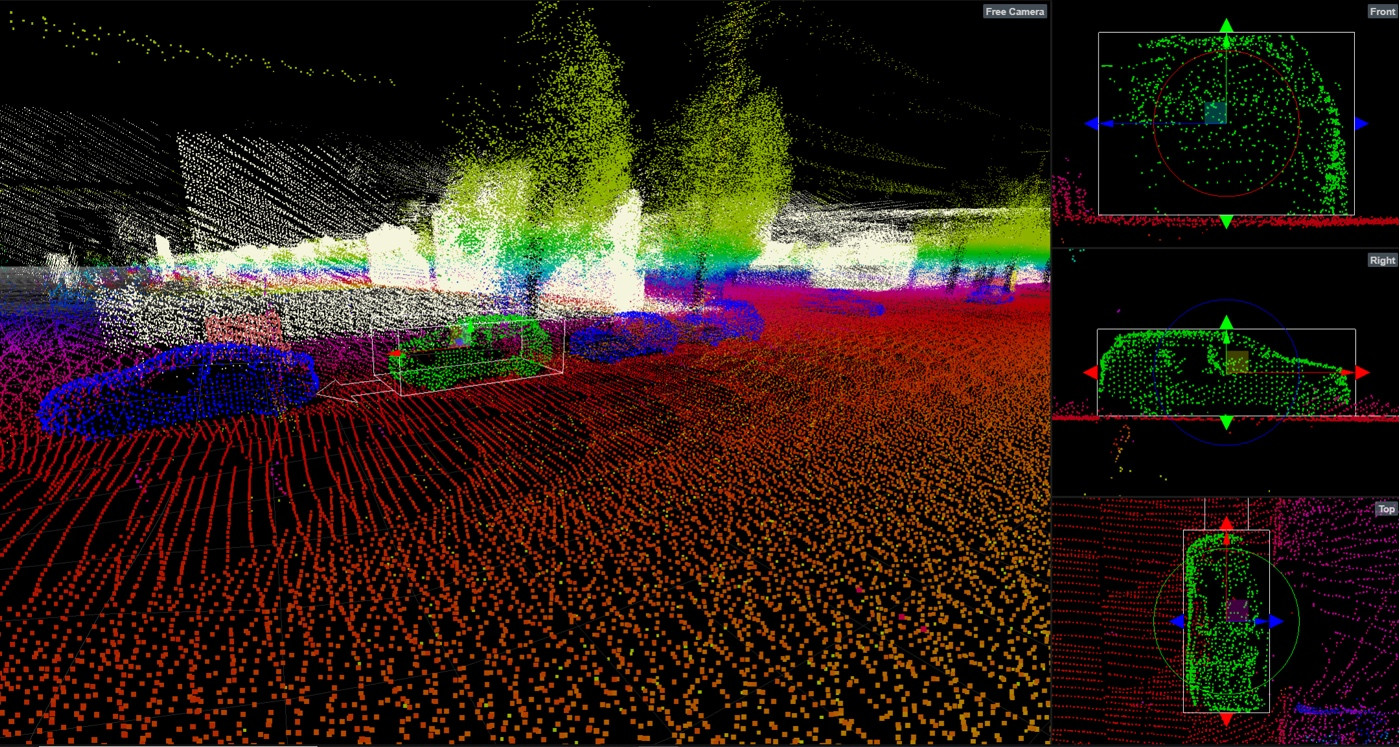
Challenges of Ground Truth Generation
Recognizing the critical role ground truth data annotations play and their significant impact on model effectiveness, the well-known principle of "garbage in, garbage out" becomes even more evident – the quality of the results depends on the quality of the input data.
However, living up to this principle doesn’t come without its challenges.
Generating reliable ground truth annotations poses challenges - including the risk of missing annotations or inconsistent interpretations. This makes the model perform poorly because it lacks a consistent and exhaustive learning signal.
In some cases, you could also come across a new example for which no labeling guidelines are provided. Or, in other scenarios, you’ll require pre-existing specialized knowledge to perform the labeling task correctly. Collaboration between domain experts and the annotation process becomes crucial for achieving precise ground truth annotations when handling these edge cases. Being aware and attentive to these challenges is key to ensuring the delivery of accurate ground truth labels.
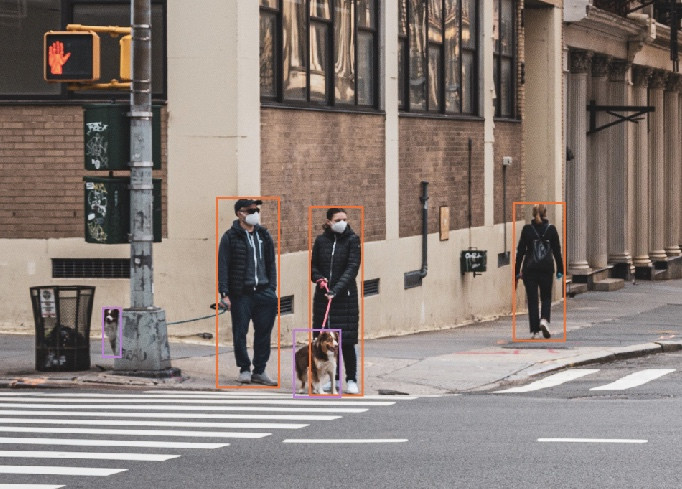
At understand.ai, we combine our ground truth annotation platform with domain expertise and AI/ML and cloud knowledge to deliver ground truth data - fast scalable and at high-quality. Check out our end-to-end solution for ground truth generation!
This article summarizes information based on contributions from UAI Staff.